Artificial Intelligence needs Human Intelligence to know anything at all
Last month, FilterLabs.AI released Talisman, our new tool that gives organizations and individuals access to hyper-local discursive and behavioral data through our powerful natural language processing and data-modeling tools. We hope you’ll check it out! In this newsletter, we want to give you a walk-through not only of some recent insights on public sentiment in Russia, but of how we used Talisman, in conjunction with human insight and perspectives, to find them.
Every day millions of new bits of information appear online. There are newspapers and magazines, local and national television stations, and internet news sites—along with an endless maze of social media, blog posts, forums, and messaging apps.
There was a time when mainstream news sources, and even online outlets, could credibly promise to make sense of the world. But now, with the overwhelming amount of information and opinion available, every effort to explain the world risks becoming just one more bit of digital noise, adding to the general cacophony.
One of the promises of Large Language Models (LLMs) is that by using them to gather and analyze enormous amounts of data, people can regain some larger sense of what’s going on. Using these models, FilterLabs can track narratives as they emerge, spread, and disappear, giving a sense of what people are thinking or feeling about an issue.
But neither LLMs nor any other AI can make sense of information themselves. On the contrary, human intelligence is necessary both to pose the questions and to make sense of the results.
Fine-tuning search queries for relevant results
For instance, for the past year, FilterLabs has been tracking Russian mainstream news and social media narratives about protests. What are people protesting? Why? And are there differences between the way Russia’s (and generally Kremlin-friendly) news outlets discuss these questions and the narratives individual Russians are discussing online?
FilterLabs often uses a lexical search parser with rich syntax capabilities to focus its investigations on narrower segments of the discourse. In this case, for example, we know that there are always protests around elections, but we thought it would be interesting to look at what else leads ordinary Russians to voice dissent.
So, we began by examining mainstream news and online social sources for stories/posts that discuss “protests” but which do not mention “elections” or related terms like “voting” or “polls.” By running a more specific search — in this case, protests − (elections OR voting OR polls) — we can narrow our focus to artifacts that are relevant to the question.
Using Talisman, we scoured thousands of social and news media sites to analyze attitudes across tens of thousands of posts. Here’s what we found.
In mainstream Russian media:
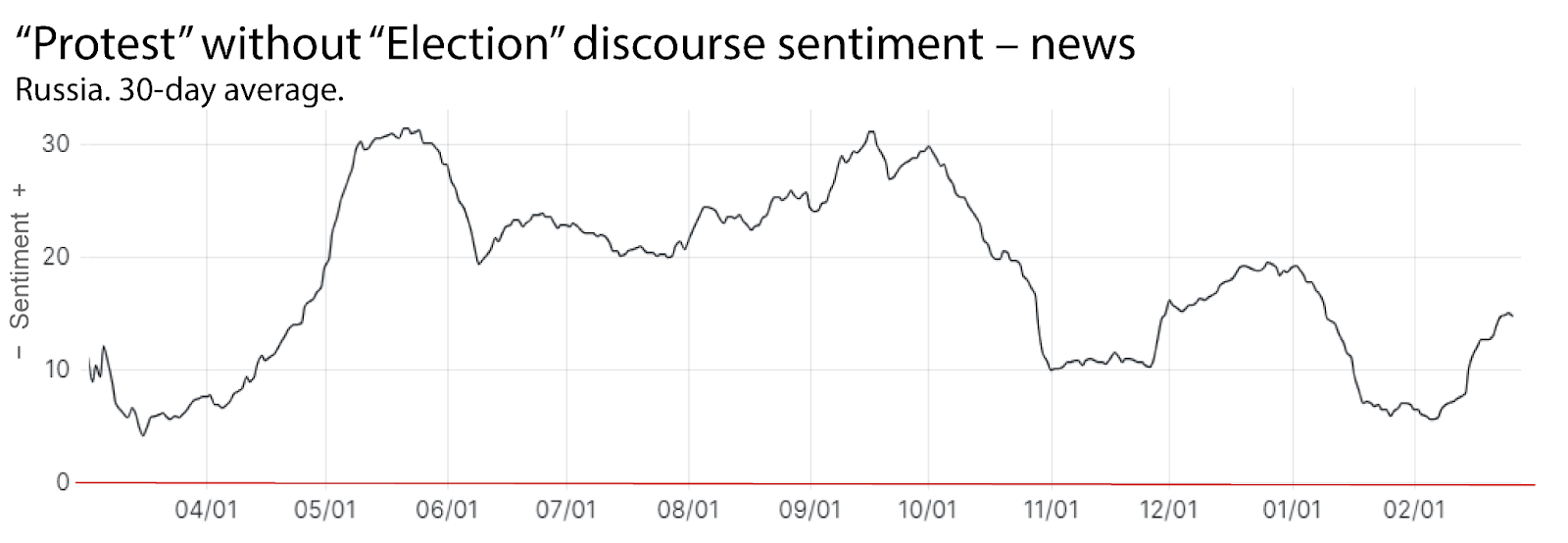
On social media (including Russian social media platforms, Telegram, and local online forums and sites):
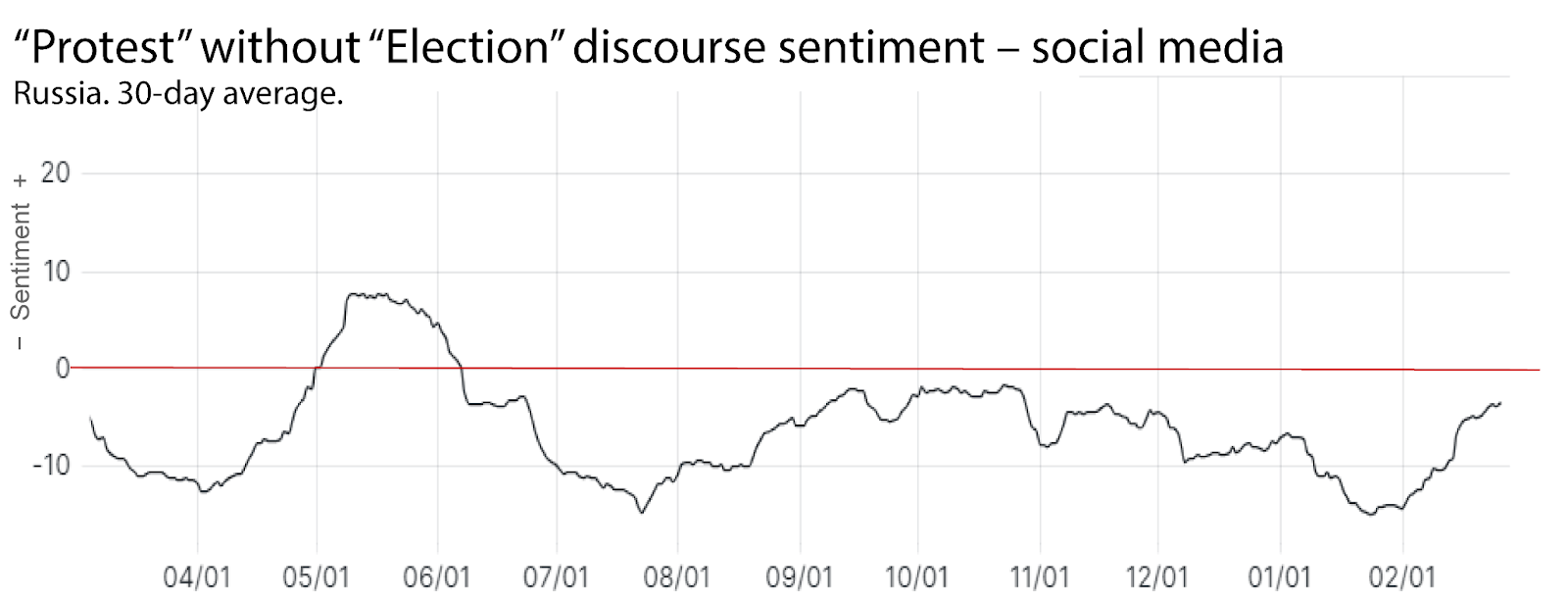
Talisman’s analysis of language in articles calculates a sentiment or attitude level in each piece of source material, the average of which is represented by the lines on the graphs above. This sentiment/attitude score is based on the amount of positive or negative language used in each post, article, or comment.
In general, the average positivity or negativity is less revealing than the moments when the line suddenly changes direction. This is usually a sign that a new story has appeared—either organically or through a government information push (i.e. a propaganda campaign).
Digging below sentiment analysis
Here is where human intelligence becomes indispensable once again. Notice the pronounced fall in the social media sentiment score between mid-May and mid-July 2023, which does not appear so dramatically in mainstream news. FilterLabs starts with a query and a sentiment analysis, but we don’t stop there. Talisman enables us to zero in on points of interest and take a direct look at the underlying data artifacts to get a sense of what is behind the shift. In this case, we found that several stories were circulating on social media during the summer downward spike:
- Opposition leaders Lilia Chanysheva received a prison sentence (“this is so scary,” said one commentator)
- Alexander Navalny’s sentence was extended
- An activist in Khabarovsk was arrested for a solo protest
- Other protestors had been arrested for defaming the Russian army
- Residents in Tbilisi protested the opening of the air border between Georgia and Russia
What can we learn from these narratives? Many Russians are genuinely concerned about political repression and the ongoing war. Beyond unfair elections, many are talking about the ongoing political oppression in increasingly negative terms. Others are recognizing, and protesting, Russia’s expanding military footprint. Furthermore, because our data is geo-located, we were able to tell that these protests were spread across the Russian empire—from Tbilisi in the west all the way to Khabarovsk, the very eastern edge.
In all of this, use of a large language model was indispensable for gathering and analyzing data on this scale, but understanding the data’s significance still requires careful interpretation—by humans.
To learn more about Talisman, visit FilterLabs.AI.